Research Supported by the NITMB
Research supported by the NSF-Simons National Institute for Theory and Mathematics in Biology focuses on developing mathematical frameworks that illuminate emergent capabilities of biological systems. We are developing the theory and mathematics needed to highlight the fundamental roles of physical, chemical, and biological constraints as organizing principles for understanding biological mechanisms. NITMB will focus on fields of mathematics where the constraints of biological systems show promise for novel developments, including geometry, topology, optimization theory, dynamical systems, high-dimensional statistics, mathematical machine learning, inverse problems, statistical inference, and stochastic processes. Understanding constraints from mathematical and biological perspectives provides a unique opportunity for interdisciplinary work, with mathematical research that will advance our knowledge of biology and biology research that will catalyze new mathematics.
Explore detailed project highlights for NITMB supported research on the Research Highlights page
A new mathematical framework for classification in cell state dynamics
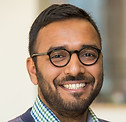
Yogesh Goyal
Assistant Professor, Cell and Developmental Biology
(Northwestern University)
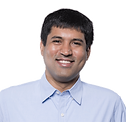
Suriyanarayanan Vaikuntanathani
Professor, Department of Chemistry
(University of Chicago)
Abstract: Our proposal emerges from a crucial challenge in cell biology: how do transcriptionally identical cells make different fate decisions when exposed to therapeutic drugs? We have observed that while cancer cells may appear homogeneous, they can develop remarkably diverse resistance trajectories when treated with drugs. To address this fundamental question, we propose developing new mathematical tools that unite concepts from statistical mechanics of deep neural networks, non-equilibrium statistical mechanics, and information theory to understand how biological networks function as classifiers. Our work extends our recent findings showing how biochemical networks' classification capacity can be systematically tuned through factors like input promiscuity. We anticipate that this ambitious undertaking will establish a mathematically consistent framework for defining cell states and their transitions, elucidate the minimal requirements for biological networks to classify perturbations, and create predictive tools for cellular responses to therapeutic interventions.
Form and Function of Drifting Olfactory Representations in the Piriform Cortex
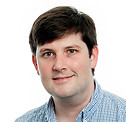
James Fitzgerald
Associate Professor, Department of Neurobiology
(Northwestern University)

Andrew Fink
Assistant Professor, Department of Neurobiology
(Northwestern University)
Abstract: Cognition and behavior are generated by patterns of neural activity. This has led many to equate brain functions with specific neural activity patterns, also called representations. However, recent data suggest that the mapping between neural representations, cognition, and behavior is more complicated. For instance, the set of neurons representing an odor’s identity in the olfactory (piriform) cortex changes over time. Such shifts in neural representations are termed “representational drift,” because they are devoid of discernible learning, forgetting, or behavioral alterations. Recent work has modeled representational drift in neural networks as the random exploration of representations that correctly produce a memorized set of input-output associations. This revealed that representational drift can benefit memory by finding sparse representations that make the system more robust to noise and continual learning. However, current models do not produce realistic representational drift, and it is unclear if this theoretical benefit occurs for biological systems. Here we will assess whether this robustness benefit applies to realistic models of representational drift in the piriform cortex. First, we will use data from the Fink Lab to quantify the geometry of representational drift and its statistics of change. Previous findings suggest that there is no linear stable subspace, so we will specifically search for nonlinear representational features that are invariant to drift. Second, we will generalize the Fitzgerald Lab’s analyses of neural network solution spaces from linear readouts to nonlinear readouts that better match the empirically stable dimensions. Finally, we will combine these results to build and analyze a representational drift model that realistically mimics the piriform cortex. This work will advance both biology and mathematics, as representational drift is a fundamental biological mechanism, and new mathematics will be needed to quantify representational geometry and neural network solution spaces.
Developing a mathematics for evolved systems
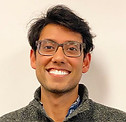
Arjun Raman
Assistant Professor, Pathology
(University of Chicago)
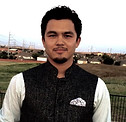
Bipul Pandey
Postdoctoral Scholar, Physics
(University of Chicago)

Benjamin A. Doran
Graduate Student
(University of Chicago)
Abstract: Systems that arise through the evolutionary process—iterative selection and variation—are qualitatively distinct from engineered systems in many ways from a functional and evolutionary standpoint. However, our capacity to understand how evolved systems manifest these differences is substantially limited because evolved systems are apparently extremely complex, comprised of many parts that interact together in unintuitive ways. Our laboratory has demonstrated that naturally evolved systems are hierarchically organized into layers of information that encode the complex whole. This result motivates creating a new mathematics that can use data collected on natural systems to generate hierarchically layered, functional emergent systems. We anticipate that our findings will lay the foundation for the mathematics of natural emergent simplicity, thereby creating generative models qualitatively distinct from those that exist today. The personnel involved in this effort are Dr. Bipul Pandey (Ph.D., Physics; Postdoctoral Scholar, University of Chicago) and Benjamin A. Doran (Graduate Student; Pritzker School of Molecular Engineering, University of Chicago).
Invasions in a Four-Species Cyclically Competing Ecological Community
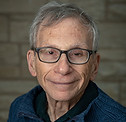
Alvin Bayliss
Professor, Engineering Sciences & Applied Mathematics
(Northwestern University)

Vladimir Volpert
Professor, Engineering Sciences & Applied Mathematics
(Northwestern University)
Abstract: We consider ecological communities consisting of 4 species engaging in cyclic competition. One can visualize the competition scheme by considering the four species, call them u, v, w, and z, as located on a clock, at 12:00 o’clock, 3 o’clock, 6 o’clock and 9 o’clock, respectively, with each species having a competitive advantage over its counterclockwise neighbor, i.e., species v (3 o’clock) wins over species u (12 o’clock) and similarly for the other competition pairs. When the competition is strong such communities are known to be dynamically stable, with two stable alliances formed by species which do not directly compete, i.e., u − w and v − z alliances , the only possible long-time outcomes of the competition. However, in nature the competitive interactions will not be the same for each species, furthermore there can be internal competition (and possibly predation) within each alliance. We consider how to determine which alliance is stronger depending on parameters of the competition. Said another way, which alliance will be able to displace the other alliance (in colloquial terms invade its territory). This problem is a version of a classical mathematical problem that transcends ecology - given two stable states of a system how to determine which state is dominant. This problem can be reduced to analysis of a system of four coupled boundary problems on an infinite interval. This system cannot be solved exactly. We will develop mathematical methods to address this problem. We will primarily employ asymptotic analysis - generally formulating the problem as a singular system, with one or more small parameters, and developing methods to approximate the solution in appropriate parameter regimes. Our analysis will enable us to determine the steady state outcome of the competition scheme, i.e., the surviving alliance.
Adaptation and Evolvability through Reinforcement Learning
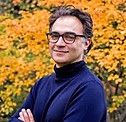
Vincenzo Vitelli
Professor, Department of Physics
(University of Chicago)
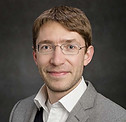
Seppe Kuehn
Associate Professor, Ecology & Evolution
(University of Chicago)
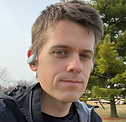
Bradly Stadie
Assistant Professor, Statistics & Data Science
(Northwestern University)
Abstract: The research proposal addresses the biological question of how the complexity of the genotype-to-phenotype mapimpacts adaptability and evolvability in dynamic environments, using reinforcement learning (RL) as a framework. The new mathematics being developed is a analytical and computational interrogation of how the complexity of the underlying network governing the behavior of an RL agent impacts its performance on learning tasks. Anticipated outcomes include theoretical insights into evolutionary dynamics, testable predictions about adaptability and evolvability, and experimental validation using microbial systems such as algae under temporally correlated light and temperature stresses
Modeling RNA sequence-structure-function relationships with multiscale higher-order graph neural networks
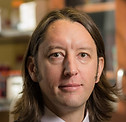
Julius Lucks
Professor, Chemical & Biological Engineering
(Northwestern University)
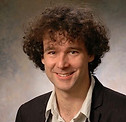
Risi Kondor
Associate Professor, Computer Science, Statistics
(University of Chicago)
Abstract: RNAs play central roles in regulating, maintaining, and defending the genomes of all organisms, with regulatory RNA sequences controlling almost all aspects of gene expression. Many of these RNA functions are linked to RNA structures that mediate interactions amongst cellular gene expression machinery, bind ligands, and perform catalysis. A central goal in biology then has been to solve the ‘RNA folding problem’ – to understand how RNA sequence determines RNA folding which governs RNA function. Once deciphered, the solution to the RNA folding problem would improve our understanding of living systems and our ability to program RNAs for biotechnologies. Graph neural networks (GNNs) are a promising new mathematical approach to modeling biomolecules, but currently do not have the mathematical properties needed to capture the features of large RNA molecules that can exist in multiple states. Here we propose to develop a new theory of graph modeling that encodes multiscale interactions within the graph architecture, preserving necessary properties of equivariance. To do so, we will derive new mathematical relationships of multiscale equivariant message passing and prove that the resulting model is the most general possible permutation equivariant multiscale neural architecture. By advancing the theory of higher order multiscale GNNs we will create a new, broadly applicable general class of neural architectures, which will apply to create a new approach to modeling RNA sequence-structure-function relationships.
Uncovering the link between chromatin organization and global transcriptional regulation

Luís A. Nunes Amaral
Erastus Otis Haven Professor, Engineering Sciences & Applied Mathematics
(Northwestern University)

Vadim Backman
Sachs Family Professor, Biomedical Engineering & Medicine
(Northwestern University)
Abstract: The goal of the proposed research is to identify and develop mathematical formulations that will enhance our mechanistic and quantitative understanding of how cell types and their idiosyncratic states can be maintained or can evolve over time or in response to stimuli. We will use experimental data to construct the multiple interaction layers — from transcript factor regulation to chromatin accessibility — regulating gene expression and will use a multiplex network formalism to model different cellular states. Our modeling will enable us to understand the processes by which, for example, a cancer state deregulates gene expression.
Characterizing excitability and its applications to immunity

Elizabeth Jerison
Assistant Professor, Physics
(University of Chicago)
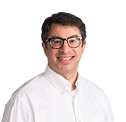_adobespark.png)
Aaron Dinner
Professor, Department of Chemistry
(University of Chicago)
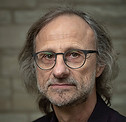
Hermann Riecke
Professor, Engineering Sciences & Applied Mathematics
(Northwestern University)
Abstract: The functions of many biological systems — including spiking neurons and activating macrophages — depend on excitable dynamics: a small perturbation triggers a rapid, nonlinear ramp followed by a return to equilibrium. While this behavior is common biologically, excitability lacks a precise mathematical definition, and the generic properties of these systems remain unclear. Prior work in theoretical neuroscience has explored in detail specific models that produce excitable behavior. This work describes the emergence of excitable dynamics near different types of bifurcations, and shows that distinct mathematical structures describe different types of neurons and their computational properties. Importantly, the same perturbation can have opposite effects depending on the mathematical origin of the excitability and the timing of the perturbation relative to the state of the system. Thus predicting and controlling the behavior of these systems depends on understanding the underlying dynamical structure, which demands enumeration of the types of excitable systems and their mathematical properties. We will take a two-pronged approach to define and classify excitable systems. First, we will extend transition path theory (TPT) to excitable systems and use it to define excitability precisely. Second, we will combine TPT with machine learning to map dynamical behavior over a broad class of models to enumerate the types of excitability. Both normal and pathological immune responses ‘flare’ — immune signaling molecules (cytokines) and immune cell populations amplify transiently before returning to baseline. This behavior suggests that systemic immune responses may be excitable. Observational data and modeling of multiple sclerosis and the existence of genetic disorders that cause spontaneous recurrent hyperinflammatory flares support this hypothesis. As in neuroscience, minimal dynamical models of these excitations could be powerful tools to understand the information processing capabilities of the immune system and develop strategies to intervene in systemic immune responses. However, we generally lack tractable experimental systems in which to make the controlled perturbations and quantitative observations necessary to test these hypotheses. To enable investigation of the excitable properties of systemic immune responses, we will study a ‘cytokine storm’ response triggered by a systemic pulse of the pathogen-associated molecular pattern lipopolysaccharide (LPS) in larval zebrafish. We will use this system to test properties such as the existence of an excitation threshold, and use our new classification of excitable systems to develop models capturing key nonlinear features of the response. Ultimately, we aim to understand which perturbations, at what stage of the response, would be necessary to return a systemic immune response to a homeostatic fixed point, allowing for the design of dynamical interventions.
Applications of free probability to the diversity of response and variability in neuronal networks

Brent Doiron
Heinrich Kluver Professor,
Neurobiology & Statistics
(University of Chicago)
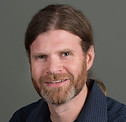
David Freedman
Professor, Neurobiology
(University of Chicago)
Abstract: Cognition is supported by neuronal activity that spans several brain regions, and understanding how this activity is coordinated is essential for a coherent theory of neuronal function. We will study how the structure of a visual categorization task in non-human primates influences the coordinated activity of three brain areas known to be essential for proper task performance. While neuronal connectivity does influence how brains perform tasks on average, a key signature of connectivity is how it also determines the fluctuations (or variability) of brain activity during tasks. We will model the trial-to-trial variability of distributed population responses with simple, yet nonlinear, recurrent circuit models. A key advance of our proposal is to consider both the input to a brain region and the wiring within and between brain regions to be randomly structured. This choice will require the use of novel analysis techniques based in free probability theory as applied to random matrices, which can untangle how two sources of randomness contribute to the variability of circuit response. Our theory will make testable predictions which can be explored in our experimental framework. In total, our work will provide a deep understanding of how diverse population brain circuitry supports the underlying mechanics of neuronal codes.